"Inferstructure" and the geopolitical dynamics of different chips.
Inferences from Minerva Technology Policy Advisors. Vol.41 - 5 December, 2024
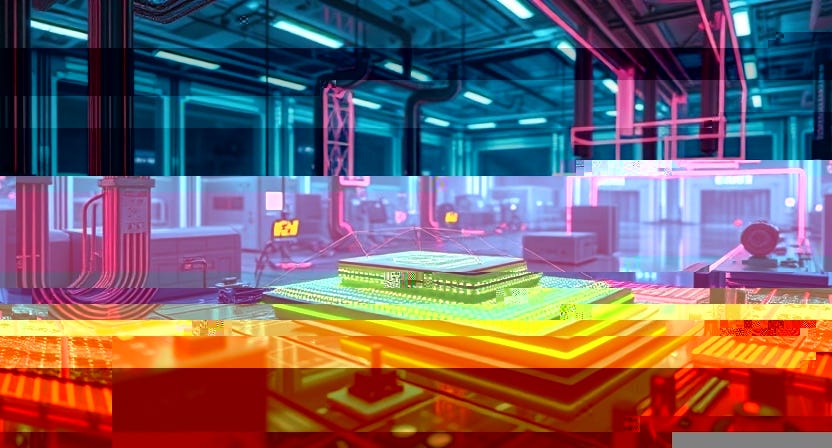
This week, one of Nvidia's closest rivals in advanced chip making—Cerebras Systems—announced results from a component that appears to run frontier models faster, and at much lower latency, than bigger competitors.
So what? The advanced chip market may be reaching a transition point as demand for specialized components and semiconductors shifts towards inferencing: capital flows, policy levers, and the balance of power in the ecosystem could shift along with it.
What is inferencing? Inference makes artificial intelligence useful, once pre-training has made it possible. It’s how a model that has been pre-trained on known data decides what to do when fed novel data. Put another way, if pre-training is where artificial intelligence goes to school, inference is where it starts doing useful work.
The market. Today, pre-training accounts for roughly 80 percent of the overall demand for compute related to artificial intelligence, while around 20 percent is used for inference. Some analysts predict that by 2027, the ratio will have flipped, with demand for inference accounting for the majority of the market. That transition could have big ramifications.
The make-up. It would mean that the make-up of useful compute resources around the world is no longer concentrated as clearly in the hands of nations that invested heavily in pre-training capabilities. GPU clusters optimized for pre-training will take up a smaller share of compute-by-market-value in data centers, and inference-specific chips running on devices, via cloud services and on-premises—much of them closer to users where applications require low latency—will take up a larger share.
The mania. Just as pre-training GPTs has seen a period of intense hype and attention, so too could inference-specific hardware. And just as soaring demand for GPUs to build pre-training clusters has fueled the booming chip sector and driven national governments to invest in “sovereign” compute capabilities for training, inference could push several chipmaker stocks that are already through the roof, into the stratosphere, as well as opening the door to new players.
Nvidia, which became the world’s most valuable company by market capitalization due to demand for its pre-training GPU clusters (and rubbed off plenty of gold dust on TSMC as Nvidia’s supplier of cutting-edge semiconductors), is still in a position to lead the move to inference-specific and blended hardware solutions including GPUs and other proprietary architectures that speed up inference.
The opportunity. Most companies aren’t running their own artificial intelligence workloads yet. While estimates of adoption of artificial intelligence tools among US companies hover around 45 percent, with most usage being experimentally based on LLMs-as-a-service, this number is set to grow as adoption gains steam. As companies build out their technology stacks to support more extensive use of pre-trained models, the demand for chips that can run end-point AI processes more quickly and efficiently will increase.
What’re the inference-specific requirements? The requirement for lower latency, energy efficiency and functionality in smaller compute substrates make the technology stack needed for inference different from pre-training. In short, inference hardware privileges memory and compute co-location to reduce bottlenecks, integrated compilers and the reduction of caches and switches.
Inferstructure... to coin a term, will also require the 5G base stations and coverage, for use on mobile. The emergence of peer-network computing for inference could mean organizations get more inference-specific workloads done with their existing devices rather than buying tokens on a third-party service.
Is it a zero-sum game? No. Just as adoption and innovation can continue in parallel, or as Jeffery Ding put it to Inferences; “you can walk and chew gum at the same time…”; demand for both pre-training GPUs and other inference-specific chips is likely to keep growing. It’s the relative share of compute for training and inference that could shift, and be delivered by a different suite of companies and economies.
What’s next? While Cerebras’ announcement is impressive, making improved compute performance for inferencing available to a broad base of users throughout the economy at a reasonable price point will remain a challenge.
Some firms are betting on capabilities that make access to a blend of chip types all at once, including GPUs, LPUs, TPUs and other accelerators, to harmonize the workloads all the way along the value chain of a large language model from training to inferencing; realising that the majority of enterprise customers will never engineer their own end-to-end artificial intelligence solutions.
The upshot? For business, inference-specific chips are likely to enter their own hype cycle; and pre-training isn’t coming to an end. Nor is demand for GPUs likely to dry up. But as large language model capabilities diffuse through the economy, the technology stack for optimizing inferencing and extracting value from model outputs in an enterprise setting will become more important.
Geopolitically, the rise of inferstructure could reignite debates about China’s role in 5G networks, while also putting pressure on the US government to further tighten restrictions on China’s access to key inference-enabling technologies. “Sovereign” compute policies that have focused almost exclusively on ensuring sufficient access to compute for pre-training, will have to adjust to account for inference too.
In the Chinese market companies have begun targeting inference efficiencies to deliver more value from models. 01.ai and DeepSeek have developed models with comparable performance on academic benchmarks, and made them much cheaper to call. 01.ai’s Yi-Lightning is a close third place behind OpenAI and Google models.
Domestically, the energy politics of the inference-intensity era—which will play out almost everywhere—will also look different than those of the pre-training era (where infrastructure was confined to huge, remote and specifically located data centers). The wider geographic footprint and embeddedness of inferstructure in the day-to-day economy will mean companies that want to build new energy capacity to deliver on enterprise solutions will need to engage with a greater variety of local communities, not all of which will be pleased about the prospect of paying higher energy rates.
These dynamics will increase the urgency in finding real, productivity-enhancing uses for artificial intelligence. If inference is where artificial intelligence gets down to useful work, it can also show ordinary people that the technology delivers real value as they go about their daily lives.
Note from Inferences: an in(fer)ception here if you will… Have thoughts on this topic, including the distribution of compute hardware and what the most significant requirements are for inference as compared to pre-training? Get in touch with us; kevin@minervapolicy.com and luke@minervapolicy.com
What we’re reading:
The fine print on Biden's third major package of semiconductor export controls.
Sinolytics’ Dr. Jost Wübbeke on China’s countermeasures.
Mohammed Soliman’s paper on The Role of the Middle East in the US-China Race to AI Supremacy.
What we’re looking ahead to:
6 - 7 February: The Inaugural Conference of the International Association for Safe and Ethical AI, Paris, France.
10 - 11 February 2025: AI Action Summit in Paris, France.
11 - 13 February 2025: World Governments Summit 2025, Dubai, United Arab Emirates.
12 February 2025: Chief AI Officer Summit UK, London.
April 2025 (expected): G7 Digital Ministerial, Canada.
2 - 4 June 2025: AI+ Expo and Ash Carter Exchange in Washington, DC.
9 - 11 July 2025: AI for Good Global Summit.